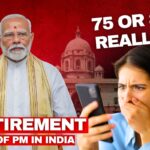
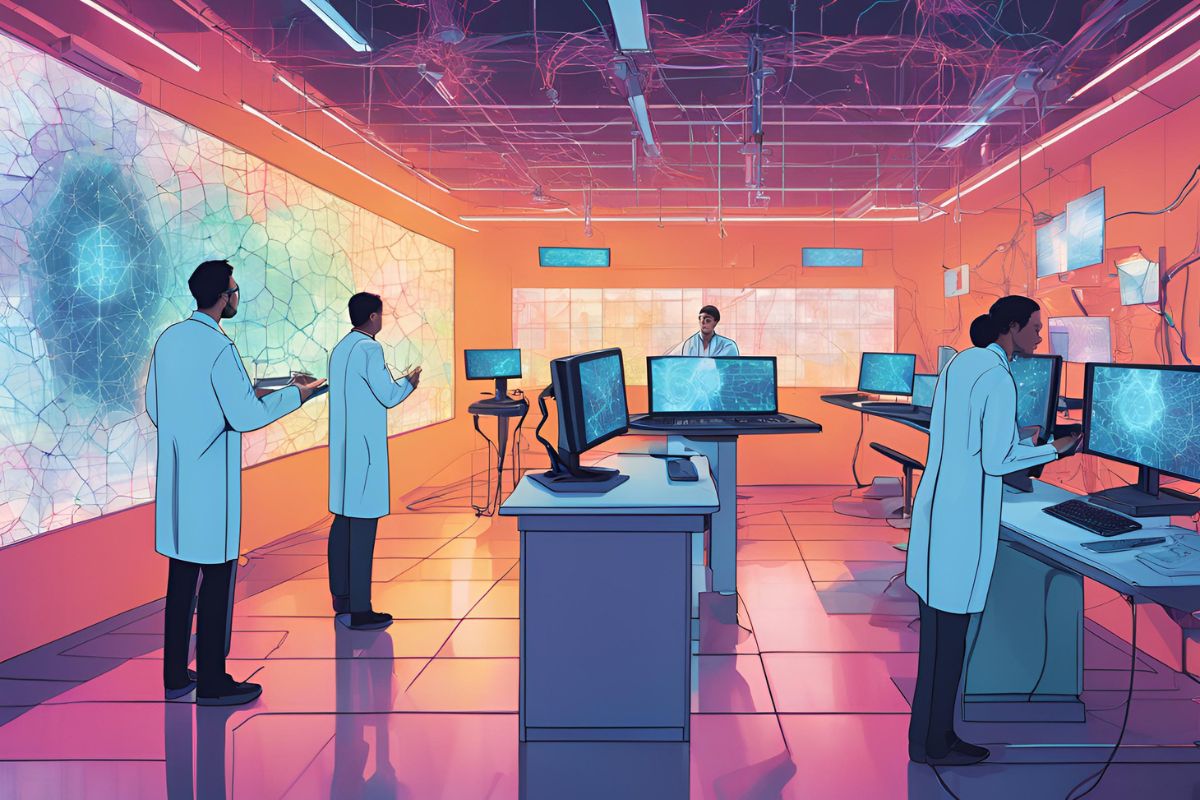
Neural networks are capable of operating at great speeds and effectiveness. They are capable of learning efficiently, and this is all possible thanks to what is referred to as XNN, or eXtreme Neural Networks. XNN is developing into a useful approach to solving real issues, the main reason being its ability to process a lot of information very rapidly. Whether it’s in healthcare, finance, or automation, XNN is helping systems make decisions faster and better. This article explores why we believe XNN is a game-changer for neural networks.
Table of Contents
ToggleExtreme Learning Machines (ELMs) focus on additional learning methods, such as classification, regression, clustering, and feature learning. Practically, they operate as a feedforward neural network (NN) characterised by an exceptional learning method whereby the hidden node parameters are fixed by random assignment and go through no adjustments in the course of training. With this approach, ELMs learn faster and generalise well as compared to conventional neural networks.
As defining features of ELMs, weights and biases of the hidden layer remain randomly assigned at all times. These are fixed once assigned in a specific range and do not change throughout the process of training. Therefore, it creates the burden of adjusting these parameters iteratively from the training phase.
By fixing the hidden layer parameters, ELMs reduce the learning problem to a linear optimisation problem; hence, it has fewer complexities in arriving at a solution. This leads to shorter training times when one compares them with other types of neural networks that have a series of repetitive processes, such as the backpropagation algorithm.
When hidden nodes are increased sufficiently, ELMs have been shown to be able to approximate any continuous function. This implies that they can perform complex data relationships; thus, they are flexible in their use for many different purposes.
Although there is some randomization in the hidden layer, most of the time ELMs perform well on test sets that were not seen during training. This is because ELMs are designed in a way that automatically locates and grasps the true representation behind the data without excessive fitting.
ELM networks can be used in many applications, such as classification, regression, clustering, sparse approximation, data compression and feature learning. Also, thanks to their flexible architecture, they can be modified for a given problem area.
The XNNs help in the diagnosis of diseases by examining the medical history and the medical images of the patients. For example, XNN helps doctors make precise diagnoses by identifying tumours in MRI scans or spotting diabetic retinopathy in retinal images.
XNNs assist in predicting future trends for assets such as stocks by recognising patterns from past records. They can recognise patterns that are useful to investors and analysts about various deals and investments.
Speech transforming into text is done via XNNs, as seen in voice-enabled devices. They allow machines to comprehend and act upon voices, making human-machine interaction more enjoyable.
Self-driving cars leverage XNNs to perform computational analysis of the data captured by electronic sensors and cameras to sight objects like pedestrians and other vehicles. This technology is important in ensuring smart road safety and instantaneous driving decision-making.
Banks and other institutions use XNNs to keep track of transactions and unearth fradulent activities. XNNs make it easy to spot transactions that deviate from the norm, thereby preventing unauthorised activities immediately.
Weather and climate forecasts are done using XNNs to analyse structured data. Sound meteorological data enables the forecasting of storms, a series of days with rainfall, as well as an increment in temperature. For instance, they alert governments and organisations to natural catastrophes such as hurricanes or floods.
In the retail sector, customers’ needs are taken into account by examining shopping patterns and preferences through XNNs. This assists the platform to suggest various products and targeted discounts to customers. This helps in recommending products or offering personalised discounts to shoppers. For example, an e-commerce store might display additional items to its customers that the customer has already seen or purchased, thereby enhancing the user experience and sales.
XNN transforms seamlessly the way we interact with technology and changes it to become quicker, smarter and more efficient in tackling specific issues. It is definitely allowing many to improve their quality of life across many key arsenals, from health care to gaming industries. XNN is also providing the key to enabling our technology-hungry future with its capability to overcome big challenges with ease and in little time.